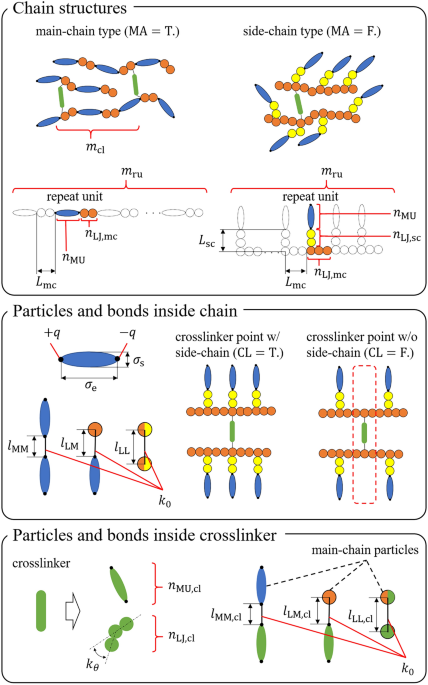
Wermter, H. & Finkelmann, H. Liquid crystalline elastomers as artificial muscles. e-Polymers 1, 013. https://doi.org/10.1515/epoly.2001.1.1.111 (2001).
Buguin, A., Li, M.-H., Silberzan, P., Ladoux, B. & Keller, P. Micro-actuators: When artificial muscles made of nematic liquid crystal elastomers meet soft lithography. J. Am. Chem. Soc. 128, 1088–1089. https://doi.org/10.1021/ja0575070 (2006).
Li, M.-H. & Keller, P. Artificial muscles based on liquid crystal elastomers. Philos. Trans. R. Soc. A Math. Phys. Eng. Sci. 364, 2763–2777. https://doi.org/10.1098/rsta.2006.1853 (2006).
Ohm, C., Brehmer, M. & Zentel, R. Liquid crystalline elastomers as actuators and sensors. Adv. Mater. 22, 3366–3387. https://doi.org/10.1002/adma.200904059 (2010).
White, T. J. & Broer, D. J. Programmable and adaptive mechanics with liquid crystal polymer networks and elastomers. Nat. Mater. 14, 1087–1098. https://doi.org/10.1038/nmat4433 (2015).
Kularatne, R. S., Kim, H., Boothby, J. M. & Ware, T. H. Liquid crystal elastomer actuators: Synthesis, alignment, and applications. J. Polym. Sci. B Polym. Phys. 55, 395–411. https://doi.org/10.1002/polb.24287 (2017).
Guin, T. et al. Layered liquid crystal elastomer actuators. Nat. Commun. 9, 2531. https://doi.org/10.1038/s41467-018-04911-4 (2018).
Urayama, K., Honda, S. & Takigawa, T. Deformation coupled to director rotation in swollen nematic elastomers under electric fields. Macromolecules 39, 1943–1949. https://doi.org/10.1021/ma052762q (2006).
Winkler, M., Kaiser, A., Krause, S., Finkelmann, H. & Schmidt, A. M. Liquid crystal elastomers with magnetic actuation. Macromol. Symp. 291–292, 186–192. https://doi.org/10.1002/masy.201050522 (2010).
Okamoto, T., Urayama, K. & Takigawa, T. Large electromechanical effect of isotropic-genesis polydomain nematic elastomers. Soft Matter 7, 10585–10589. https://doi.org/10.1039/C1SM06372C (2011).
Corbett, D. & Warner, M. Changing liquid crystal elastomer ordering with light—a route to opto-mechanically responsive materials. Liq. Cryst. 36, 1263–1280. https://doi.org/10.1080/02678290903062994 (2009).
Kato, T. & Tanabe, K. Electro- and photoactive molecular assemblies of liquid crystals and physical gels. Chem. Lett. 38, 634–639. https://doi.org/10.1246/cl.2009.634 (2009).
White, T. J., Serak, S. V., Tabiryan, N. V., Vaia, R. A. & Bunning, T. J. Polarization-controlled, photodriven bending in monodomain liquid crystal elastomer cantilevers. J. Mater. Chem. 19, 1080–1085. https://doi.org/10.1039/B818457G (2009).
Lee, K. M. et al. Photodriven, flexural-torsional oscillation of glassy azobenzene liquid crystal polymer networks. Adv. Funct. Mater. 21, 2913–2918. https://doi.org/10.1002/adfm.201100333 (2011).
Gelebart, A. H., Mulder, D. J., Vantomme, G., Schenning, A. P. H. J. & Broer, D. J. A rewritable, reprogrammable, dual light-responsive polymer actuator. Angew. Chem. (Int. Ed. Engl.) 56, 13436–13439. https://doi.org/10.1002/anie.201706793 (2017).
White, T. J. Photomechanical effects in liquid crystalline polymer networks and elastomers. J. Polym. Sci. B Polym. Phys. 56, 695–705. https://doi.org/10.1002/polb.24576 (2018).
Bispo, M., Guillon, D., Donnio, B. & Finkelmann, H. Main-chain liquid crystalline elastomers: Monomer and cross-linker molecular control of the thermotropic and elastic properties. Macromolecules 41, 3098–3108. https://doi.org/10.1021/ma7026929 (2008).
Ohm, C., Haberkorn, N., Theato, P. & Zentel, R. Template-based fabrication of nanometer-scaled actuators from liquid-crystalline elastomers. Small 7, 194–198. https://doi.org/10.1002/smll.201001315 (2011).
Fleischmann, E.-K., Ohm, C., Serra, C. & Zentel, R. Preparation of soft microactuators in a continuous flow synthesis using a liquid-crystalline polymer crosslinker. Macromol. Chem. Phys. 213, 1871–1878. https://doi.org/10.1002/macp.201200276 (2012).
Shenoy, D. K., Laurence Thomsen, D. III., Srinivasan, A., Keller, P. & Ratna, B. R. Carbon coated liquid crystal elastomer film for artificial muscle applications. Sensors Actuators A Phys. 96, 184–188. https://doi.org/10.1016/S0924-4247(01)00793-2 (2002).
Thomsen, D. L. et al. Liquid crystal elastomers with mechanical properties of a muscle. Macromolecules 34, 5868–5875. https://doi.org/10.1021/ma001639q (2001).
Urayama, K., Kohmon, E., Kojima, M. & Takigawa, T. Polydomain–monodomain transition of randomly disordered nematic elastomers with different cross-linking histories. Macromolecules 42, 4084–4089. https://doi.org/10.1021/ma9004692 (2009).
Burke, K. A. & Mather, P. T. Soft shape memory in main-chain liquid crystalline elastomers. J. Mater. Chem. 20, 3449–3457. https://doi.org/10.1039/B924050K (2010).
Tsuchitani, A., Ashida, H. & Urayama, K. Pronounced effects of cross-linker geometries on the orientation coupling between dangling mesogens and network backbones in side-chain type liquid crystal elastomers. Polymer 61, 29–35. https://doi.org/10.1016/j.polymer.2015.01.069 (2015).
Rešetič, A. et al. Stress-strain and thermomechanical characterization of nematic to smectic a transition in a strongly-crosslinked bimesogenic liquid crystal elastomer. Polymer 158, 96–102. https://doi.org/10.1016/j.polymer.2018.10.049 (2018).
Herbert, K. M. et al. Synthesis and alignment of liquid crystalline elastomers. Nat. Rev. Mater. 7, 23–38 (2022).
Yakacki, C. et al. Tailorable and programmable liquid-crystalline elastomers using a two-stage thiol-acrylate reaction. RSC Adv. 5, 18997–19001 (2015).
Brannum, M. T. et al. Deformation and elastic recovery of acrylate-based liquid crystalline elastomers. Macromolecules 52, 8248–8255. https://doi.org/10.1021/acs.macromol.9b01092 (2019).
Skačej, G. & Zannoni, C. Molecular simulations shed light on supersoft elasticity in polydomain liquid crystal elastomers. Macromolecules 47, 8824–8832. https://doi.org/10.1021/ma501836j (2014).
Whitmer, J. K., Roberts, T. F., Shekhar, R., Abbott, N. L. & de Pablo, J. J. Modeling the polydomain-monodomain transition of liquid crystal elastomers. Phys. Rev. E 87, 020502. https://doi.org/10.1103/PhysRevE.87.020502 (2013).
Tagashira, K., Takahashi, K., Fukuda, J. & Aoyagi, T. Development of coarse-grained liquid-crystal polymer model with efficient electrostatic interaction: Toward molecular dynamics simulations of electroactive materials. Materialshttps://doi.org/10.3390/ma11010083 (2018).
Doi, H., Takahashi, K. Z., Tagashira, K., Fukuda, J.-I. & Aoyagi, T. Machine learning-aided analysis for complex local structure of liquid crystal polymers. Sci. Rep. 9, 16370. https://doi.org/10.1038/s41598-019-51238-1 (2019).
Takahashi, K. Z., Aoyagi, T. & Fukuda, J.-I. Multistep nucleation of anisotropic molecules. Nat. Commun. 12, 5278 (2021).
Yasuoka, H., Takahashi, K. Z., Fukuda, J.-I. & Aoyagi, T. Molecular architecture dependence of mesogen rotation during uniaxial elongation of liquid crystal elastomers. Polymer 229, 123970 (2021).
Yasuoka, H., Takahashi, K. Z. & Aoyagi, T. Trade-off effect between the stress and strain range in the soft elasticity of liquid crystalline elastomers. Polym. J. 54, 1017–1027 (2022).
Takahashi, K. Z. Molecular cluster analysis using local order parameters selected by machine learning. Phys. Chem. Chem. Phys. https://doi.org/10.1039/D2CP03696G(in press).
Kularatne, R. S., Kim, H., Boothby, J. M. & Ware, T. H. Liquid crystal elastomer actuators: Synthesis, alignment, and applications. J. Polym. Sci. B Polym. Phys. 55, 395–411 (2017).
Cercignani, C. The Boltzmann equation. In The Boltzmann Equation and Its Applications, 40–103 (Springer, 1988).
Einstein, A. Über die von der molekularkinetischen theorie der wärme geforderte bewegung von in ruhenden flüssigkeiten suspendierten teilchen [adp 17, 549 (1905)]. Ann. Phys. 14, 182–193 (2005).
Hammett, L. P. Some relations between reaction rates and equilibrium constants. Chem. Rev. 17, 125–136 (1935).
Rogers, D. & Hopfinger, A. J. Application of genetic function approximation to quantitative structure–activity relationships and quantitative structure-property relationships. J. Chem. Inf. Comput. Sci. 34, 854–866 (1994).
Héberger, K. Quantitative structure–(chromatographic) retention relationships. J. Chromatogr. A 1158, 273–305 (2007).
Katritzky, A. R. et al. Quantitative correlation of physical and chemical properties with chemical structure: Utility for prediction. Chem. Rev. 110, 5714–5789 (2010).
Le, T., Epa, V. C., Burden, F. R. & Winkler, D. A. Quantitative structure–property relationship modeling of diverse materials properties. Chem. Rev. 112, 2889–2919 (2012).
Jiao, Z., Escobar-Hernandez, H. U., Parker, T. & Wang, Q. Review of recent developments of quantitative structure–property relationship models on fire and explosion-related properties. Process Saf. Environ. Prot. 129, 280–290 (2019).
Williams, M. L., Landel, R. F. & Ferry, J. D. The temperature dependence of relaxation mechanisms in amorphous polymers and other glass-forming liquids. J. Am. Chem. Soc. 77, 3701–3707 (1955).
Doi, M., Edwards, S. F. & Edwards, S. F. The Theory of Polymer Dynamics Vol. 73 (Oxford University Press, 1988).
Wallin, K. The master curve method: A new concept for brittle fracture. Int. J. Mater. Prod. Technol. 14, 342–354 (1999).
Flory, P. J. Principles of Polymer Chemistry (Cornell University Press, 1953).
Stevens, M. P. Polymer Chemistry Vol. 2 (Oxford University Press, 1990).
Hiemenz, P. C. & Lodge, T. P. Polymer Chemistry (CRC Press, 2007).
Stanton, D. T. & Jurs, P. C. Development and use of charged partial surface area structural descriptors in computer-assisted quantitative structure-property relationship studies. Anal. Chem. 62, 2323–2329 (1990).
Cruciani, G., Crivori, P., Carrupt, P.-A. & Testa, B. Molecular fields in quantitative structure–permeation relationships: The Volsurf approach. J. Mol. Struct. THEOCHEM 503, 17–30 (2000).
Couling, D. J., Bernot, R. J., Docherty, K. M., Dixon, J. K. & Maginn, E. J. Assessing the factors responsible for ionic liquid toxicity to aquatic organisms via quantitative structure–property relationship modeling. Green Chem. 8, 82–90 (2006).
Liu, W., Lu, H., Cao, C., Jiao, Y. & Chen, G. An improved quantitative structure property relationship model for predicting thermal conductivity of liquid aliphatic alcohols. J. Chem. Eng. Data 63, 4735–4740 (2018).
Huan, T. D. et al. A polymer dataset for accelerated property prediction and design. Sci. Data 3, 1–10 (2016).
Audus, D. J. & de Pablo, J. J. Polymer informatics: Opportunities and challenges. ACS Macro Lett. 6, 1078–1082 (2017).
Ramprasad, R., Batra, R., Pilania, G., Mannodi-Kanakkithodi, A. & Kim, C. Machine learning in materials informatics: Recent applications and prospects. npj Comput. Mater. 3, 1–13 (2017).
Mannodi-Kanakkithodi, A. et al. Scoping the polymer genome: A roadmap for rational polymer dielectrics design and beyond. Mater. Today 21, 785–796 (2018).
Jackson, N. E., Webb, M. A. & de Pablo, J. J. Recent advances in machine learning towards multiscale soft materials design. Curr. Opin. Chem. Eng. 23, 106–114 (2019).
Aoyagi, T. Deep learning model for predicting phase diagrams of block copolymers. Comput. Mater. Sci. 188, 110224 (2021).
Morita, H., Miyamoto, A. & Kotani, M. Recoverably and destructively deformed domain structures in elongation process of thermoplastic elastomer analyzed by graph theory. Polymer 188, 122098 (2020).
Aoyagi, T. High-throughput prediction of stress–strain curves of thermoplastic elastomer model block copolymers by combining hierarchical simulation and deep learning. MRS Adv. 6, 32–36 (2021).
Aoyagi, T. Optimization of the elastic properties of block copolymers using coarse-grained simulation and an artificial neural network. Comput. Mater. Sci. 207, 111286 (2022).
Berardi, R., Zannoni, C., Lintuvuori, J. S. & Wilson, M. R. A soft-core Gay–Berne model for the simulation of liquid crystals by Hamiltonian replica exchange. J. Chem. Phys. 131, 174107 (2009).
Breiman, L. Random forests. Mach. Learn. 45, 5–32. https://doi.org/10.1023/A:1010933404324 (2001).
Pedregosa, F. et al. Scikit-learn: Machine learning in python. J. Mach. Learn. Res. 12, 2825–2830 (2011).
Wu, Z. et al. Moleculenet: A benchmark for molecular machine learning. Chem. Sci. 9, 513–530 (2018).
Ware, T. H., McConney, M. E., Wie, J. J., Tondiglia, V. P. & White, T. J. Voxelated liquid crystal elastomers. Science 347, 982–984. https://doi.org/10.1126/science.1261019 (2015).
Guo, Y., Zhang, J., Hu, W., Khan, M. T. A. & Sitti, M. Shape-programmable liquid crystal elastomer structures with arbitrary three-dimensional director fields and geometries. Nat. Commun. 12, 1–9 (2021).
Zhang, J. et al. Liquid crystal elastomer-based magnetic composite films for reconfigurable shape-morphing soft miniature machines. Adv. Mater. 33, 2006191 (2021).
Xiao, Y.-Y., Jiang, Z.-C., Hou, J.-B. & Zhao, Y. Desynchronized liquid crystalline network actuators with deformation reversal capability. Nat. Commun. 12, 1–10 (2021).
Aoyagi, T. et al. A general-purpose coarse-grained molecular dynamics program. Comput. Phys. Commun. 145, 267–279. https://doi.org/10.1016/S0010-4655(02)00271-0 (2002).